ARM TECHNOLOGY
What is "ARM"?
ARM stands for Adaptive Reasoning Model. In the Nirvana Club, we have taken
ARM several levels above OmniTrader through the use of applied Artificial Intelligence.
- Overview
- arm 2
- arm 3
- arm 4
- ARM 5
- ARM 6
- Deep Learning
The History of ARM2
ARM 2 Release 2 was our first version of ARM Technology delivered to Club members. Our ARM was based on a Genetic Algorithm process home brewed here at Nirvana. ARM 2 R2 was tunable by the user in terms of desired signal accuracy vs. signal count - a major advancement.
Neural Network was added in ARM2 Release 3 to determine a positive or negative score at any bar in a chart. The network in ARM2 R3 determines a "likely up" or "likely down" state for the chart at any bar. This gave us a new capability, since ARM2 R3 can be applied to ANY trading signal.
In 2002 and 2003, we began experimenting with pattern recognition concepts. ARM2 R4 is based on these findings, which are an application of J.M. Hurst's work on Valid Trend Lines. The proprietary Nirvana Club
(VTL-B) System is still being used to improve performance in ARM3.
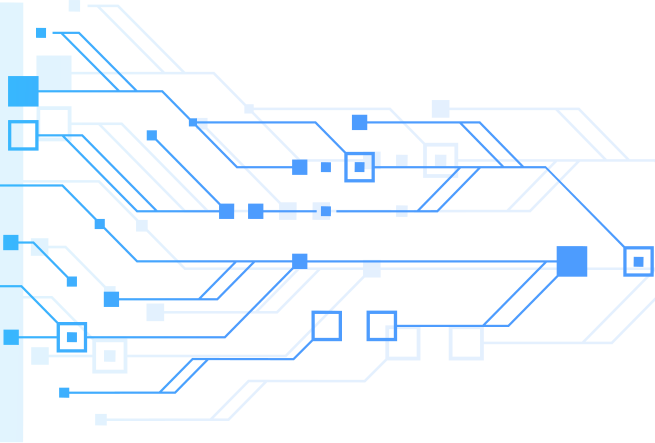
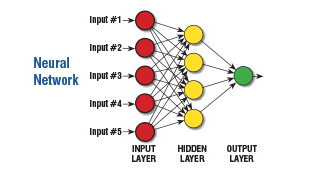
ARM2 R3 was developed using a Neural Network, and is capable of signal accuracies of 65% - 70%
ARM3- The Next Generation
The version of ARM Technology, called ARM3, was literally 'heads and shoulders' above the prior versions. Using advances in Artificial Intelligence and taking advantage of faster processors available, we rebuilt our Neural Network and Genetic Algorithm components from the ground up.
Members can now build their own Knowledge Bases quickly and easily by simply training our Networks or GAs on their own symbol lists. Or, they can create their own networks using knowledge they have about Technical Analysis. And, in 2006, we improved the Genetic Algorithm component further.
The Move to Equity Curves
Before 2007, we had been using a signal measurement technique called Next Pivot Points, to measure Signal Accuracy. This method told us whether the Entry Signals on a Strategy were improved, but did not yield strategies which could be "mechanically" traded because there were no actual Exits.
All that changed in 2006. Our Artificial Intelligence technology is now powerful enough to apply real exits to our strategies - producing Strategies that can be traded mechanically.
Our focus now is on improving performance in the Equity Curves until we have reached our Ultimate Trading Machine goal (see About the Club). However, since we are delivering Strategies to Members that show mechanical profitability over long periods of time, many members are engaging the market with these products
NSP-33 NN
In 2008, we launched a new mechanical strategy called NSP-33. This strategy showed the ability to generate an average return of 20% per year from 1998 through July 2009.
Bull Market or Bear Market – this amazing strategy has consistently pumped out profits year after year. After the Strategy was delivered to the Nirvana Club, we applied our ARM3 Neural Network to it to create NSP-33 NN.
The result was another immediate performance improvement. By August 2009, NSP-33 NN was up an incredible 38%.

A Breakthrough In Artificial Intelligence - ARM4
In 2009, we pushed the envelope ahead again, with the new Consensus Block. This new A.I. tool makes it possible to measure the predictive power of any list of formulas or indicators. This is very important, because we are able to eliminate the ones that don’t work well and focus on those that do.
When we began this research, we were hoping to build a tool to assist us in this task. What we ended up with was so powerful, we increased our ARM technology to ARM4.
The first Strategy to be developed with ARM4 is NSP-35 CB (Consensus Block). It is based on a careful selection of indicator formulas that identify the best trading situations. Our Consensus Block identifies those ranges that are most predictive, and then it combines them into a “consensus vote”.
The power of this approach is evident in the NSP-35 CB Equity Curve, showing over 30% annual profit from 2001 through 2009.
Improving Strategies with ARM4
In 2010, we added Data Mining to our Neural Network process, which dramatically improved performance. In many cases we are seeing the profits DOUBLE in our best Strategies!
We are using our ARM technology to empower traders to achieve a superior return. As we do this, we will continue to build new Strategies with tor ARM technology. With the performance gains we are currently seeing, this could easily be the year that we hit our Ultimate Trading Machine goals.
- About ARM2
- Arm2 charts
- arm2 r4
- 13th Tab

In this chart, you can see multiple ARM2 signals firing in the
direction of trend.
About ARM2 R3
ARM2 was designed around the concept of relating Market Factors (such as the current retracement percentage for a move) with Clues (e.g., volume increasing) to predict the accuracy of a given Signal using Artificial Intelligence methods.
After launching the Nirvana Club in 1996, we built a Genetic Algorithm to find combinations of Factors and Clues that formed "rules" in a Knowledge Base, such as "If MACD is rising and Volume is increasing and today's Open is above yesterday's Close, there is a 70% chance of the security going up."
This process involved many thousands of processing hours. We networked all our machines together and let them "cook" each night to find these rules.
In 1998 we released the first Knowledge Bases to members, calling it ARM2 Release 2 (Release 1 was experimental). ARM2 R2 improved accuracy by about 10 points. That was a great start, but we had a way to go. So we continued looking for better ways to do the same job.
ARM R3 - Our First Neural Network
In 1999 we released ARM2 R3 using a commercial Neural Network. We discovered that Neural Networks are better suited to our problem (though we later re-invented the Genetic Algorithm component when we created ARM3.) Market Factors and Clues were fed as "inputs" into the Neural Network to find relationships in the data.
At each Signal event, a Neural Network was consulted to determine the likelihood of the security rising or falling based on the trained Neural Network. The Network issued a "Thumbs Up" or "Thumbs Down" indication on each Signal. Signals were then either passed to the Vote Line or not based on this determination.
The ARM2 Release 3 Neural Networks increased the accuracy of OmniTrader's buy/sell signals by 10 to 20%. An OmniTrader profile that generated an average Forward Test accuracy of 55% could generate accuracies on the order of 65 to 70 percent. This was a phenomenal achievement so early in the Club's life.

ARM2 worked extremely well on stocks that exhibit smooth changes in trend.
Note: ARM2 is no longer used by members (since ARM3 is more accurate), but we have preserved it on our Nirvana Club web site for members to download and experiment with, at their option.
How Is ARM2 Different?
An important aspect of all ARM2 (and ARM3) strategies is the fact that optimization is not used. Standard OmniTrader profiles (like Default) work by optimizing every Symbol individually to find the best parameters for the Systems being used. This is a good way to tune a System, but has the disadvantage that as soon as a new Back Test is run, the Signals will change as new parameters are chosen.
With ARM2 and ARM3, System Parameters are fixed and do not vary, which in turn means the signals will not change. Signals that are passed to the Neural Network either "make it" to the Vote Line or filtered out the same way regardless of how when the Back Test is run. The same Signal will always be seen in the Chart. This behavior definitely makes it easier to "trust" the Signals in real trading.
The Next Generation ARM2
This was very important in our understanding of how to apply Artificial Intelligence technology to the market problem. Using this knowledge, we developed the next generation of A.I. tools for members that takes advantage of OmniTraders' Strategy Builder paradigm - ARM3. ARM3 is our current Nirvana Club Signal Technology.
ARM2 R3 Charts
The Charts on this page were generated by ARM2 Release 3. We have replaced ARM2 with ARM3 in our work for the Nirvana Club. These charts were captured shortly after ARM2 Release 3 was delivered to members (May 2003.) More recent charts are available in the ARM3 section.

ARM2 did a great job of firing signals at key reversal points. You can see the excellent Short signals in December and January in this example of ADIC.

Another stock, Aeroflex with ARM2 signals firing at excellent opportunity points to go Long and Short in the first four months of 2003.

ATMI generated more signals at key opportunity points.

Here, we see ATML forming a saucer bottom in March and April (and firing a Long at the right side of the chart). Again, note the powerful Short opportunity identified in early December of '02.

AVID Technology had several nice swings in the January through March timeframe, before the outstanding Long signal which was generated in mid-March.

Beasley tends to move in nice runs once it gets going.

Here we see a number of reasonable entry points on Benchmark (however, we would probably avoid this stock due to its extreme volatility).

Biosite is a very nice trading stock, due to the smooth moves it tends to exhibit. Here you can see a great Long in December, followed by a waterfall Short in late January 2003 - all ARM2 Release 3 signals.

Continental is another smooth mover. We had some very nice opportunities to make money on this low-priced stock in late December and mid January, before volatility increased in March.

Right away, we see the fabulous Long generated on Chiron in early March (following a nice Short from mid-January).

More great signals on Chattem.

Digimarc had several nice swings from December to March.

Dionex Corporation is a volatile stock that we would probably not trade. However, a we did see a VERY profitable Long generated in January 2003.

We picked up a nice, long trend in Drugstore.com from February, after a shorter, but very profitable Long in late December.

EDS had a waterfall decline in January, which ARM2 alerted us to. It then recovered in a big way in March. Note the ARM2 signal at almost the exact bottom.

Valid Trend Lines are essentially sloping support and resistance lines, as shown here on AGN.

Here, we see Valid Trend Lines forming across swings in AIG.

Citigroup shows shorter swings, with good lines.
About ARM2 R4
ARM2 Release 4 is based on ARM2 Release 3, with the addition of Valid Trend Lines (VTLs) to boost accuracy and performance. This technique provided a SUBSTANTIAL boost in performance.
What is a Valid Trendline?
Valid Trend Lines are essentially tight lines drawn across price action, with specific factors measured to create the best possible lines, such as correlation, angle of line, break bars for the line, and so on.
To the right, you can see a few examples of this method. Valid Trend Lines essentially form sloping support/resistance lines that are very powerful.
When a strong line is violated, the odds of a move following the break are higher, leading to some of the most highly confirmed reversal signals possible.
Using Valid Trendlines to confirm ARM2 Signals.
The Valid Trend Line system is a powerful "confirmer". When used in conjunction with ARM2, it helps to isolate those signals which are firing at key reversal points and have additional, favorable characteristics - resulting in greatly increased accuracy, as described below.
Valid Trend Lines can be added to just about any reversal (swing) strategy to improve it. Many Nirvana Club members are experimenting with various hybrid concepts, and posting great results to the Nirvana Club Forums daily.
ARM2 Release 4 Accuracy
With the introduction of ARM2 Release 4, Club Members were able to establish the accuracy they want to see in their signals for the first time.
Valid Trend Lines have a setting called "Correlation". This setting defines how well the lines fit the data in the chart. By simply cranking up the value of this setting, you can get more and more accurate signals out of ARM2. This was an important advancement that led to the creation of ARM3.
Here are some performance reports from ARM2 confirmed with Valid Trend Lines, on the S&P 100 over 500 bars. It is important to note here that ARM2 profiles are not optimized - you will get the same Signals whether you look at Forward Test or Back Test results. ARM2 Release 4 Signals were typically 70%-80% accurate. *
S&P 100
Accuracy = 74% * correlation at .90 ▼

Accuracy = 82% * correlation at .99 ▼

Tuning Performance
You can see from these performance numbers that, as accuracy increased from 74% to 82%, profit per trade doubled. However, the number of trades decreased to 1/10 the initial value. Some users will want more signals. For them, the former setting is preferable. Others will want the highest accuracy possible, and run thousands of symbols through the system. For them, the latter setting is preferable.
ARM3 Evolution
ARM2 R4 was released in 2003. In 2004, we wanted to increase signal count while maintaining accuracy. This was achieved by creating multiple Knowledge Bases and Neural Networks that are tuned to specific indicators and systems.
ARM3 improved on the ARM2 Release 4 concept by making available the Neural Network Scores and a "cutoff" setting. Users can do the same kind of performance turning with ARM3 that they did in ARM2 Release 4, by adjusting the cutoff setting in the Strategy. See ARM3 for complete information.
* We measured accuracy differently for ARM2 than ARM3. For a detailed description of how we measured the accuracy of ARM2, please see How Accuracy is Measured.
- About ARM3
- NN Score
- GA Signals
- 13th Tab
What is ARM3?
We have seen many technological advances since ARM2 was created in 1996-98. Computers dramatically increased in both speed and memory. With the introduction of Trade Plans in OmniTrader 2004, we were able to create strategies from building blocks. We rewrote our A.I. engine, creating components which are collectively called "ARM3."
Two Powerful New Technologies Make it Happen.
NN Score

The new NN Score component uses a Neural Network to score signals from any system or vote combination, essentially replacing the Advisor Rating. The PRIMARY input to the NN Score component is a Signal Line within an OmniTrader Strategy - that is, a line populated with trading signals. The SECONDARY inputs, which are used for training, can be any trading system(s) and any indicator or difference between indicator or price values, such as Close(Today) - Close(Yesterday).
The component takes the inputs at points the given Signals occur, and creates a Neural Network to optimize a given target, such as "Profit 5 bars in the future." A Score is generated based on "fitness" of the inputs to the given target. Within the component, the Score can be set to a threshold, so that only the best signals are sent on to the next step in the Strategy (typically Orders, as shown above).
GA Signals
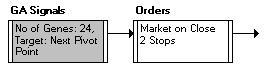
GA Signals is similar to NN Score, in that it uses internal inputs defined from technical measurements and trading systems. However, GA Signals does not score an existing signal line - it CREATES signals from the inputs. GA Signals is useful in situations where you are not sure which system to use to generate a signal, but have an idea that several systems and measurements, when combined, could create a good entry.
That is, by combining 3 Systems and 3 Indicators (for example), some combination thereof would generate a signal - you just don't know what that combination is. GA Signals iterates on the problem space using a Genetic Algorithm process, until it has converged on numerous "rules" which are then added to a Knowledge Base, such as "Long Signal when MAC-M fires a signal and Volatility is between 2.5 and 3.5 and Close is more than 5% above the 21 period Moving Average."
Advantages of this new technology
- Much higher accuracy and profitability. We are seeing Networks and Knowledge Bases with over 80% accuracy and high profit-per-trade numbers. We have also generated several mechanical strategies which use mechanical exits, creating 100% mechanical systems.
- Improve the Scores and Performance of any existing system. If you have a system you really like in OmniTrader, the NN Score component is likely to make it better. By using the "canned" settings in the component, you can turn it loose in a strategy on virtually any system and get higher Hit Rates and APRs from the same system.
- Users can Train or Retrain Networks and Knowledge Bases. If you have a list of stocks or futures you would like to "tune" ARM3 to, just activate the ToDo List and specify "ReTrain" in a profile that has ARM3 Strategies. The Knowledge Bases or Networks will be retrained on YOUR data.
- Members can experiment with their own theories. It takes minutes to define the measurements and inputs for a Neural Network or Genetic Algorithm Knowledge Base inside the NN Score or GA Signals components. If you have an idea about a combination of systems and measurements (indicators) that you believe would predict the market well together, set up an experiment, build a Knowledge Base and test it out. You have the power.
NN Score

The PRIMARY input to the NN Score component is a signal line within an OmniTrader Strategy - that is, a line populated with trading signals. The SECONDARY inputs, which are used for training, can be any trading system(s) and any indicator or difference between indicator or price values, such as Close (Today) - Close (Yesterday).
The component takes the inputs at points the given signals occur, and creates a Neural Network to optimize a given target, such as "Profit 5 bars in the future." A Score is generated based on "fitness" of the inputs to the given target. Within the component, the Score can be set to a threshold, so that only the best signals are sent on to the next step in the Strategy.
Here are some statistics on the Volatility Breakout System, before using the Neural Network enhancement, and after. You will see that the overall hit rate for the profile went fro 69% to 82%, and many unprofitable signals have been eliminated.
Virtually any system in OmniTrader can be improved the same way. We are using NN Score to boost the performance of ARM3. Clearly, we are very excited about this new technology!
Performance Reports (S&P)

BEFORE using the Neural Network

AFTER using the Neural Network
BEFORE using the Neural Network
AFTER using the Neural Network














NN Score improves virtually any trading system to generate fewer, better Signals!
GA Signals

GA Signals are useful in situations where you are not sure which system to use to generate a signal, but have an idea that several systems and measurements, when combined, could create a good entry. That is, by combining 3 Systems and 3 Indicators (for example), some combination thereof would generate a signal - you just don't know what that combination is.
GA Signals iterates on the problem space using a Genetic Algorithm process, until it has converged on numerous "rules" which are then added to a Knowledge Base, such as "Long Signal when MAC-M fires a signal and Volatility is between 2.5 and 3.5 and Close is more than 5% above the 21 period Moving Average."
Test case #1: Futures Knowledge Base on Currencies
We generated a Knowledge Base by using continuous contracts on currencies going back 30 years (that is, our Back Test for training was set to use all 30 years of data). We reserved a one-year Forward Test at the end of training as an "out of sample" test period:
Currencies Used:
Euro Dollar
British Pound
Australian Dollar
Canadian Dollar
American Dollar
Japanese Yen
Swiss Frank
After training, we changed the Back Test to one year so we could compare similar time frames (one year Back Test and one year Forward Test). The resulting performance report is shown here:

Here is a chart for the Swiss Franc:

Test Case #2: Knowledge Base for Gains
Here is a chart for Wheat:

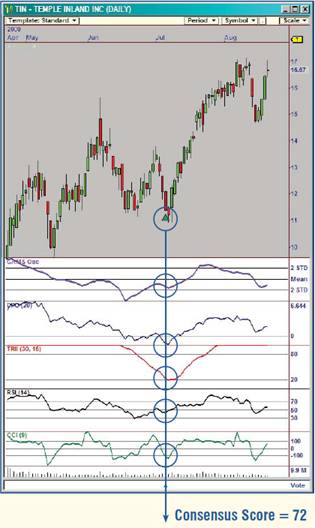
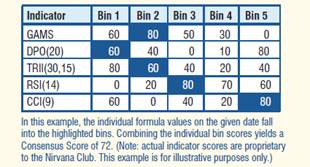
ARM4 is based on the concept of measuring technical inputs and feeding them into a Neural Network to find profitable relationships. This process has yielded some of our best strategies, including NSP-33 NN. However, one of the challenges in building these strategies has always been, “How do we identify the best inputs to use?” This year, we set about answering this question.
The Consensus Block represents a major advancement in technical analysis. It’s the perfect marriage of artificial and human intelligence, providing detailed insight into how different indicators and events help to predict future market behavior.
The Consensus Block performs two separate jobs, the first of which is called “Data Mining.” For this step, we define a list of indicators and formulas that we think might be predictive. Then we enter them in the Consensus Block and let it measure them across a large symbol list and years of data.
The result is a table that indicates the predictability of each input (see example) for different ranges of the input. In the second step, the Consensus Block combines the given inputs using a Genetic Algorithm, resulting in a score from 0 to 100. We only trade those signals with the highest scores.
With the Consensus Block, we can now greatly accelerate our development of profitable trading strategies. The fi rst is NSP-35 CB (discussed next).
The "Rocket Science" (How it Works)
The following example demonstrates how the various features of the Consensus block can be used to gain knowledge into predictive measurements and create a trading strategy. As previously discussed, several configurations are possible. In order to prove the concept, we will employ the simplest configuration that consists in a strategy with only two blocks.
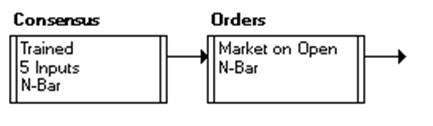
Step 1: Data Mining
First, we select a comprehensive number of measurements that - we think - may characterize the behavior of the symbols in our list. For the present example, we are using the SP100 in the daily timeframe. The following dialog box shows some of the variables that were selected. Note how some measurement formulas are repeated with different parameter values, usually the Period parameter in order to see whether a slower or a faster indicator is more predictive. For sake of simplicity, no conditions were specified in this example.
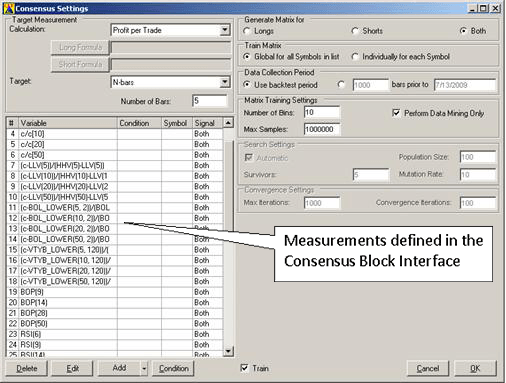
A total of 51 variables were specified in this Consensus block. In order to reduce the number of variables to a smaller set of effective measurements, we will start by performing a Data Mining run (with Perform Data Mining Only checked in the dialog box). In addition, because we intend to collect data at every bar for several years (from 2001 to 2006) and over about 100 symbols, we increase the Max Samples setting to 1,000,000, thus ensuring that all collected data samples are represented in the statistical results. The Data Mining run can now be initiated. For a large experiment such as the one described, this run can take several hours, depending on the computer system's specifications.
When the run is completed, we access the Data Mining and Matrix Analysis interface in order to identify the measurements that showed a significant correlation with the recorded target. The next figure, taken from the Consensus Block Analysis screen, depicts the relationship between CCI(9) and the average target value. With the exception of Bin(1), a well-defined decreasing function is clearly visible. After reviewing quartile and standard deviation measurements in the statistics table, we can safely say that – for this particular setup – CCI(9) has predictive value.
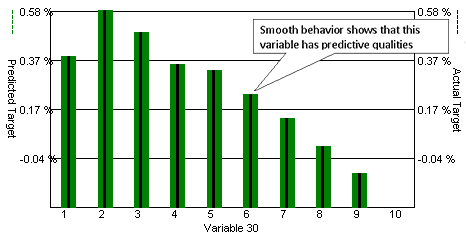
Target Sensitivity plot for CCI(9).
However, the next view shows Close / Close ten bars ago (C / C[10]) without significant correlation. This variable can be deleted from the strategy to simplify the configuration in preparation for a full training session.
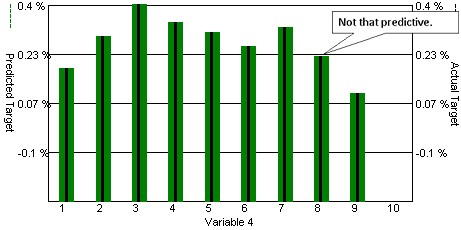
After repeating this analysis for all variables, we selected the smallest number that exhibit the best correlation with the targets:
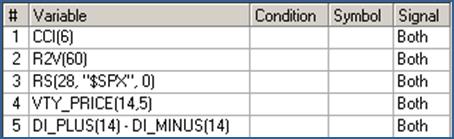
Step 2: Evaluating the Results
After we identify the indicators and other formulas we want to use for trading, we train again on ONLY those values, allowing the Consensus Block to combine individual values to produce a Consensus Score after it finishes its Data Mining Step. Consensus Scores vary from 60 to 100.
By increasing the Consensus Cutoff and running profitability tests on the resulting signals, we see a DEFINITE RELATIONSHIP between CONSENSUS SCORES and PROFITS.
The following diagrams depict the number of trades and average profit per trade results obtained by varying the Consensus Cutoff (Filter) value, the back-test is the same period used for data mining runs, while the forward-test is the remaining data up to July 7th, 2009. You can see from the graph that the profit per trade increases while the number of trades decreases at higher cutoff values, confirming that the chosen variables can indeed produce valuable predictions for the 5-bar price movement.
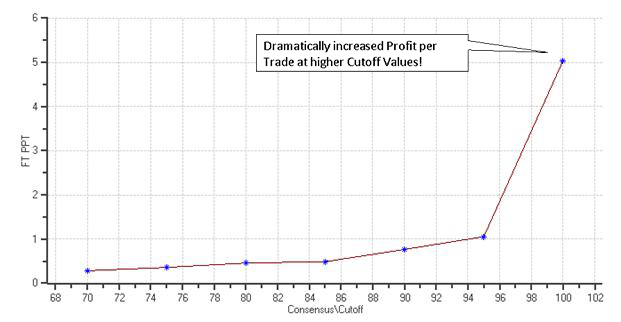
Forward-test Profit per Trade vs Consensus Cutoff
This plot not only proves that the Consensus Block “works” – it shows greatly increased profits at high Cutoff Values. The next plot shows the expected relationship between Cutoff Values and Trade Count – the higher the Cutoff, the fewer trades “pass” to the Vote Line. But, the great thing about the Consensus Block is, even at high Cutoff levels (like 96) there are still a very large number of trades.
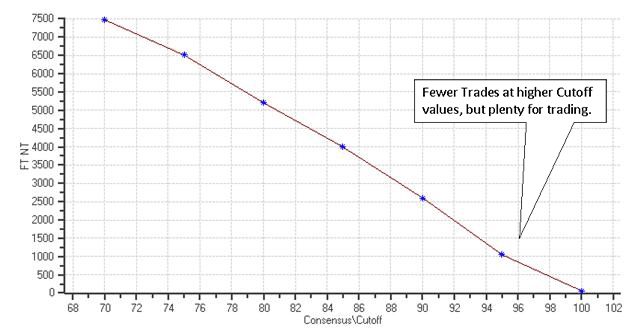
Number of Trades in the Forward Test vs. Consensus Cutoff Value
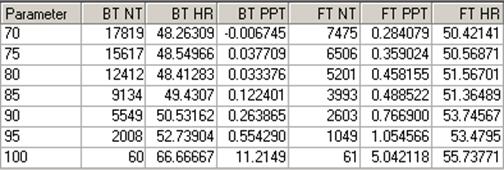
Full Statistics for different Consensus Cutoff values.
Step 3: Trading with the Results
The next screenshot shows how the consensus block in this strategy was able to identify the bottom of General Dynamics Corp on 3/9/2009 (in the forward-test period) by combining the predictions obtained by each of the indicators displayed in the chart. Trades like this are common in the output of the new NSP-35 CB Strategy, to be released to the Nirvana Club in the Fall of 2009.
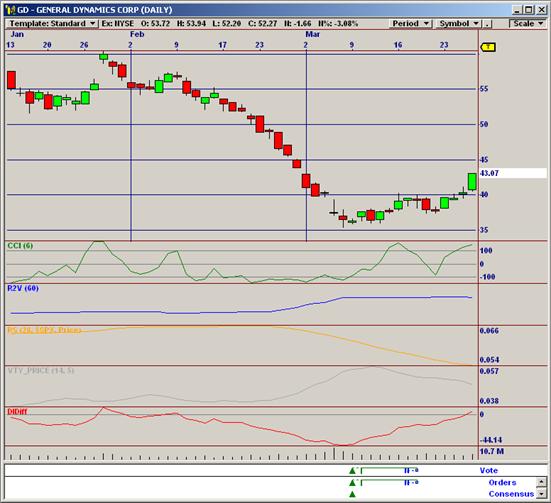
A Consensus Block trade on General Dynamics Corp. The chart
including plots for the indicators used by the Consensus Block.
In 2010, we added Data Mining to our Neural Network process, which dramatically improved performance. In some cases we are seeing the profits DOUBLE in our best Strategies!
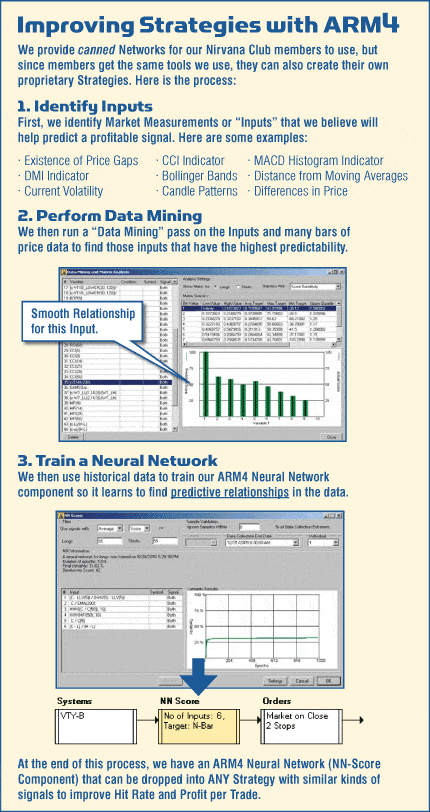
Millions have been invested in the development of ARM4, which is by far the most powerful Artificial Intelligence technology ever created for traders. As a result of recent advances, we are now seeing 80% Hit Rates in many of our Strategies— the first performance goal for The Ultimate Trading Machine.
New A.I. Technology for 2017
and Beyond!
In addition to the Neural Network another powerful tool developed in the Nirvana Club is our Genetic Algorithm.

Neural Networks combines inputs in a "fluid" way, a Genetic Algorithm is based on RULES. A Rule is a collection of factors (inputs) which indicate a strong profit when present together.
As Traders, we would like to evaluate 50, 100 or even 200 different indicators to "figure out" which ones combine to create a profitable outcome. That's a VERY large problem and it's what the G.A. excels at!
The example to the right is an actual Rule in our GA Funds Strategy. This rule was 81% Accurate with 2.84% Profit per Trade with 148 occurrences over historical data. The Knowledge Base was created over about 15 hours, and has a total of 3,500 rules.



Finding Profitable Rules in MILLIONS of bars of data
You may be wondering how the Genetic Algorithm can get such great results. The picture to the right represents 6 inputs (real problems can have hundreds.) Our first step is to measure all inputs across all our data. Inputs to the GA can be:
- Technical Indicators
- True/False Comparison
- System Signals
Multiple inputs make up a "chromosome". In the picture to the right, each chromosome has 6 inputs each. Each input will have a starting value, like "RSI(14) > 30 = True". Some inputs are set as "X" for Don't Care, which means their value is not used in that chromosome.
Each chromosome is then tested for profitability across ALL the data, recording average accuracy (“Hit Rate”), average profit per trade (“PPT”), and other statistics for each“match”.
Now we start a “genetic” process of crossover and mutation to hopefully find better results,and the process is repeated many times.Finally, the best Rules are saved to the Knowledge Base.


Over 80% Accurate!
All Nirvana Club Members have access to the ARM5 Strategies listed on our web site, including the one shown here, called "GA Funds". This Strategy , developed for trading Exchange Traded Funds (ETFs), is showing 80% accuracy in out-of-sample data. This is just one of several breakthrough Strategies developed with the G.A. in 2016.
A New Level of Profitablity
In 2016, we dramatically improved our Genetic Algorithm using techniques described onthe next page.
These advances have made it possible for us to build several outstanding new Strategies that shatter prior records, including GA Stocks RTM, shown on the right.
This Strategy has dramatically out-performed the market for the past 13 years, making an incredible 30% per year on average. Strategies this powerful are not available anywhere else.
And it gets better...
This is just one of the Strategies we released with the latest version of ARM5. I personally applied this technology to create an improved Secret Sauce Strategy as well as an ARM5 Liquid Stocks Strategy,the first one I’ve seen showing 85% profitability!


Achieving Unprecedented Accuracy & Profits
Creating a Mechanical Strategy that is 70% accurate is not easy,unless you have ARM5!
I personally trained a Knowledge Base, using the G.A., on 20very liquid stocks and was able to get out-of-sample, forward test accuracy of 85%. Then, I applied this Knowledge Base to a new list of 300 stocks, which shows nearly 75% accuracy on this expanded list. A Portfolio Simulation and recent trade list is shown to the right.
Several key improvements were developed and tested to improve the G.A. in 2016, including:
- Incremental Training
- Uniqueness of Rules
- Technical Filters
- Group-Based G.A.'s
- Boolean Imputs
- And more. . .
Each of these advancements is described in detail on the Nirvana Club Web site.The most significant were Incremental Training and the use of Filters when training a Knowledge Base with the GA.

NEW Incremental Training. This chart illustrates the Incremental process. Multiple Knowledge Bases are created so all trades are "out of sample".
Incremental Training: All Trades are in "Out of Sample Data"!
When Incremental Training is applied, the program uses a prior Training Period to create a set of rules for the NEXT TRADING Period. In this way, all trades are generated in "Out of Sample" data. So every trade you see i sone you would have seen on the right edge.

By restricting training to only occur in Trending Zones, the resulting Knowledge Base will be much more robust – finding trading opportunities when this specific condition exists.
Filters: Creating More Accurate Knowledge Bases
Another important feature we added to the G.A. in 2016 is the concept of Filters, like the Trend Filter shown here. Rules are only be generated when the Trending Filter is True, resulting in a much better and more accurate rule set. The Filter concept was used in the creation of the GA Stocks RTM Strategy.
ARM5 is the result of hundreds of steady improvements made to our A.I. technology since the Nirvana Club was founded in 1996. There is no question about it, The Club has the best and most profitable Artificial Intelligence technology in the world, making a Nirvana Club Membership the best investment you can make.
Announcing ARM6...
The Genetic Algorithm has 20+ major enhancements that were implemented from December 2018 through July of 2019. If you are simply using ARM6 Strategies, you will appreciate how much faster the Signals are generated, and how much better the Back Test and Forward Tests match.
Do you develop? Many of the enhancements benefit those who want to create the best possible Genetic Algorithm.
The speed of operation is about 100x faster, bringing time to execute 10,000 iterations down to just 20 minutes.
The new Equity Curve Fitness Function provides the best metric for comparing Rules, since it simulates trading performance of each Rule.
In Training, the Inputs and Rules are automatically managed. Automatic Binning make it easier to define “Super Inputs” that can lead to phenomenally profitable Rules, assisted by the new Internal Validation feature. Finally, users can experiment with the new SETI project to retrieve and test Inputs from our servers, and change the way the GA works by changing experimental settings in the INI file.
MAJOR SPEED IMPROVEMENTS
There are two areas of the Genetic Algorithm that require a lot of CPU processing time: Training and Signal Generation. Multi-threading on multiple cores was successfully applied in these crifical areas for dramatic speed increases.
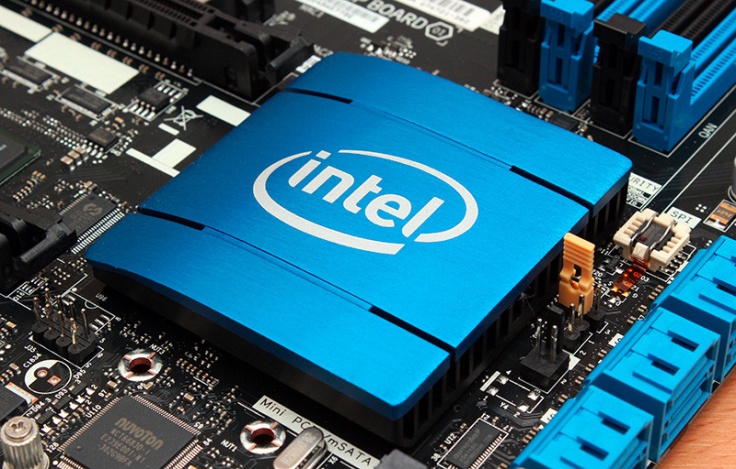
Modern CPUs have multiple Processor Cores
Multi-Core Training
The Genetic Algorithm Training code was enhanced so that multiple cores can be used to achieve parallelism.
The GA works by creating 1,000 random Rules, and then measuring each Rules across all the data so statistics can be calculated (Hit Rate, PPT, etc.) This used to be done one Rule at a time. Now many can simultaneously be measured on multiple CPUs (cores) on the computer.
Prior to the enhancement, the GA would produce new Rules at a rate of about 1 Rule every 3 Seconds. Now, it can produce 10 Rules per Second.
Multi-Core Signal Generation
At Signal Generation time, each bar of data must be evaluated on all Rules in the Knowledge Base to see which Rules are generating a Signal on that bar. This used to be done one bar at a time. Now, many Rules can be evaluated simultaneously, using separate cores of the CPU.
On Knowledge Bases with many Rules (30,000+) it used to take up to a minute to generate Signals on a single Symbol. Now, the same KB can generate Signals in 2-3 seconds – speeding it up by a factor of 30 to 50 times.
EQUITY CURVE FITNESS FUNCTION
A Rule with high Hit Rates can have low Profit per Trade values. Similarly, a Rule with a large Profit per Trade can have very low Hit Rates. A better way to compare Rules is to measure the approximate profitability of each Rule.
Equity Curve Fitness
Equity Curve is a new Fitness Function that measures Gains & Losses for each Rule to determine which Rules have the best Trading Performance.
This is done by running a quick simulation on all Hits for a Rule to arrive at an average profit percentage per bar. The effect of having more trades active at the same time is taken into account by the simulation.
Max Positions
Specifies the maximum number of trades that can be open during the simulation. This helps ensure that Rules with a large number of trades are not automatically inflated, since not all trades can be taken in a live account.
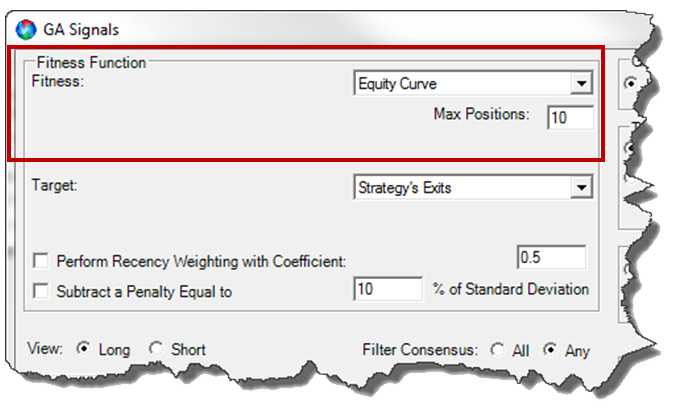
TRAINING ENHANCEMENTS
How the Genetic Algorithm works.
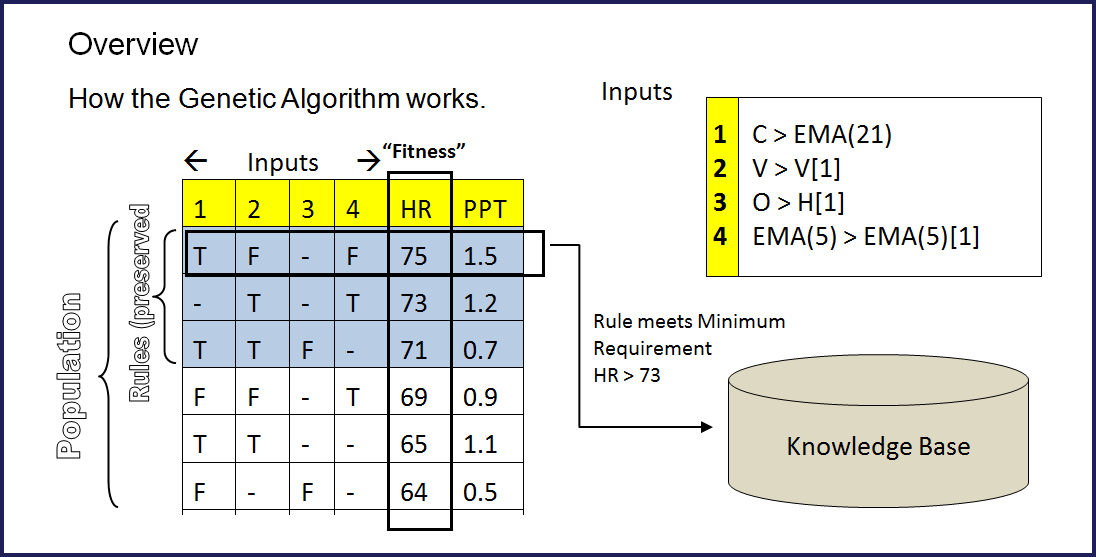
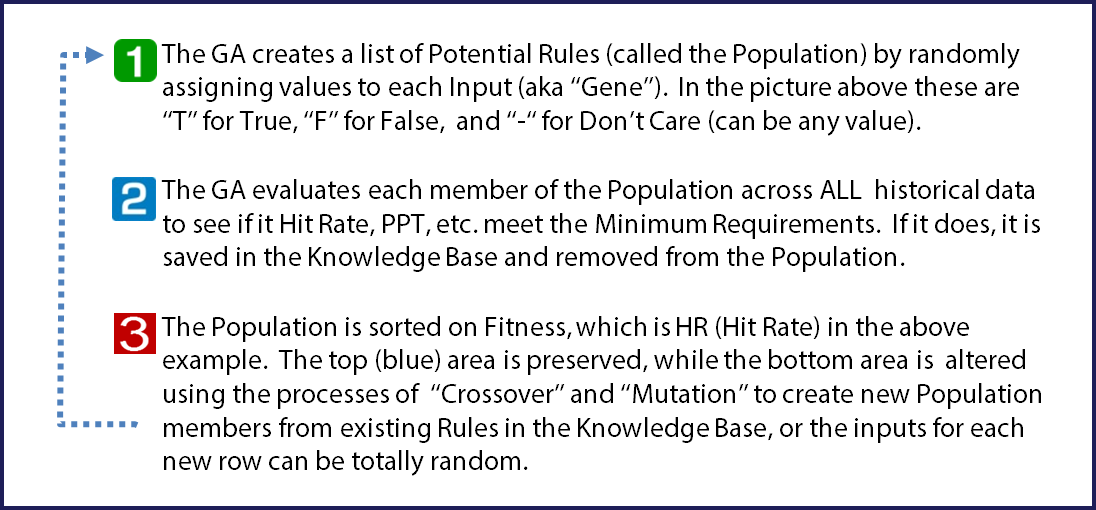
Automatic Training Constraints
The [x] Automatic check box on Minimum Requirements allows the GA to automatically increase Profit per Trade, Hit Rate, and or Min EC to encourage it to find better and better Rules. It can temporarily reduce Minimum Requirements to “jump start” the process, but will only save Rules that meet the Minimums.
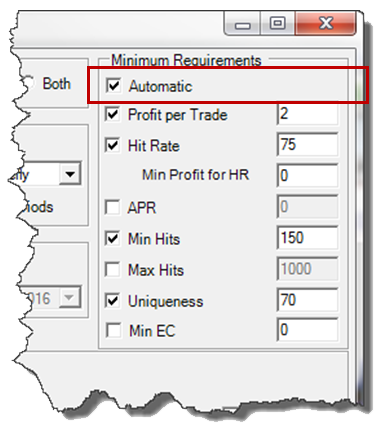
Automatic Input Reduction
Whenever the GA sees an Input that has a Delta Fitness of 0 for a given Rule, the Input will be removed from the Rule before the Rule is saved. Using this approach, Inputs that are “kept” in any Rule are the ones that make a difference in that Rule. This has the effect of creating simpler, better rules.
Manual Input Reduction
If we measure Delta Fitness for ALL Rules for an Input, we end up with an average value for how much the Rules are affected if we remove this Input. This is called Mean Delta Fitness and is shown in the Input Table under Settings.
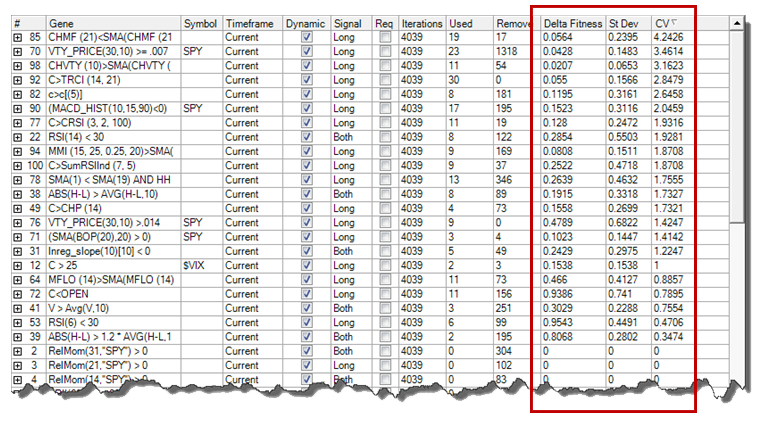
Table in Settings showing Delta Fitness Statistics for each Input
Continue Training after Deleting or Adding Inputs
In prior releases of the GA, if any inputs were deleted or added, the GA would have to be re-trained. This is no longer the case. If an Input is deleted, all Rules that have that Input will be deleted. But those that do NOT have the Input will be preserved.
This means you can delete Inputs and/or Copy/Paste Inputs from other GA’s and Continue Training, to keep getting a better and better Knowledge Base.
Automatic Rule Reduction
We have seen Knowledge Bases grow to 50,000 Rules. This causes processing to slow because every Rule must be evaluated on every bar at Signal Generation time. One of the ways we address this is by eliminating duplicate Rules.
We discovered that when two Rules have virtually identical Statistics (Hit Rate, Number of Trades, and Average PPT) they are almost always measuring the same thing, even though their Inputs are different. By deleting these Rules we avoid saving Rules to the Knowledge Base that are substantially identical.
Internal Validation
We always train in a “Back Test” period. However, if we reserve data within the same period for validation, we can tell if a Rule we have discovered is consistently valid. We use two kinds of internal validation, “Symbol” and “Time Period” validation.For Time Period Validation with 1 Validation Period, the Training Segments and Validation Segments will be the same number of bars. In the illustration to the right, 30 bars was used. When the GA is training, it looks for Rules across ALL of the Training Segments at the same time. When a valid Rule is found, it then measures occurrences and performance of the Rule in the Validation Segments.
For Time Period Validation, we have found that it is best if the number of bars used for validation is greater than 20 to avoid training and validating occurrences of a Rule that are too close together and therefore not independent.
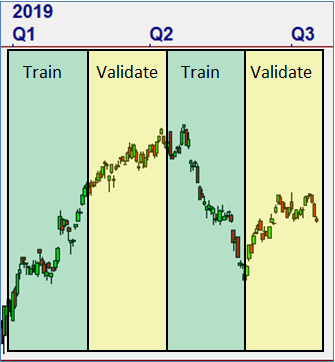
Time-Based Internal Validation with 1 Validation Period of 30 bars
Lock Trained Dynamic Periods
A feature was added a few years ago to train new Knowledge Bases on an interval, such as Quarterly.
We never want Signals to change in a Knowledge Base we are using, but if we are developing them, we may want to be able to Continue Training on the prior segments before trading with the KB.
Using this setting means we can CONTINUE Training on the Segments, which is useful to continue making them more effective. As we do this, Signals will change because more rules are generated. Once we release the Knowledge Base(s), we would no longer want to train the older segments to avoid changing Signals, so we check the “Lock trained dynamic periods” setting.
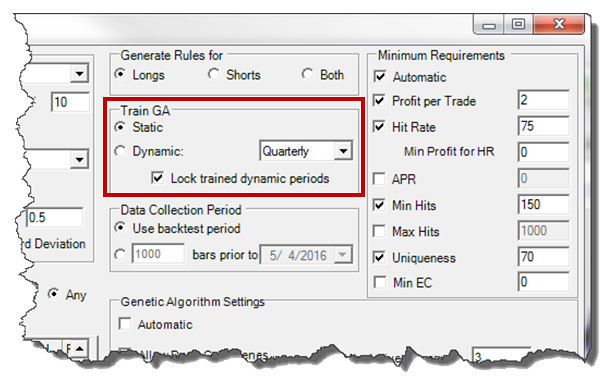
THE SETI PROJECT
In the 80’s, scientists wanted to accelerate the search for life in the universe. One idea was to “divide” the sky into many thousands of parcels and engage individuals to contribute their computers to examine the data in each parcel. This was the foundation of SETI, which stands for the “Search for Extra Terrestrial Intelligence.”
At Nirvana, SETI stands for the “Search for Extra Terrific Inputs.” SETI (a) allows individuals to invent new indicators or relationship metrics and upload them to a Server, and (b) others to download the Inputs to test and use.
How SETI Works:
When enabled, at the start of each Training, Inputs with the lowest Fitness (equal to the “Work Space” setting) are removed and replaced with inputs from the server. The INI file variable ExploratoryPercent controls this. If ExploratoryPercent is “80”, then 80% of the Inputs will be pulled randomly from the Server, and 20% will be those with the highest values of Delta Fitness (matching the Fitness Function used in the GA) on the Server.
When Training is resumed in another run of the ToDo List, Inputs are replaced again. Note that SETI is still experimental, and performance can drop as a result of Input Replacement.
Preserving Inputs
Often, we design Inputs that we want in the GA, even if they have lower Delta Fitness values. For example, we may add indicators on the market that are used rarely but effectively keep us out of Long trades if the market is weak. In this case, the protection offered by these inputs is more important than how they perform.
There is a feature in the GA that allows us to “preserve” inputs from being swapped out by SETI.
Deep Learning
A Powerful Addition to the Nirvana Club’s A.I. Arsenal
What is Deep Learning?
Deep Learning is based on a Neural Network that uses “Hidden Layers” to help it learn chart relationships.
But you don’t need to understand how it works. Just add the Deep Learning block to your Strategy and let it improve your Signals.
Trade the Best Signals Every Day!
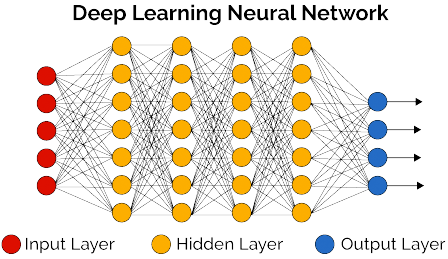
Improves Existing Strategies
When added to an existing Strategy, the Deep Learning Module will examine each Signal and determine which factors in the Market are present on the BEST Signals. The result is you get Signals with much higher accuracy and profitability – just by adding Deep Learning!
We applied Deep Learning
to our MS-22 Strategy.
Every aspect of the Strategy was improved:
• Higher Profits
• Lower Draw Downs
• Smoother Performance
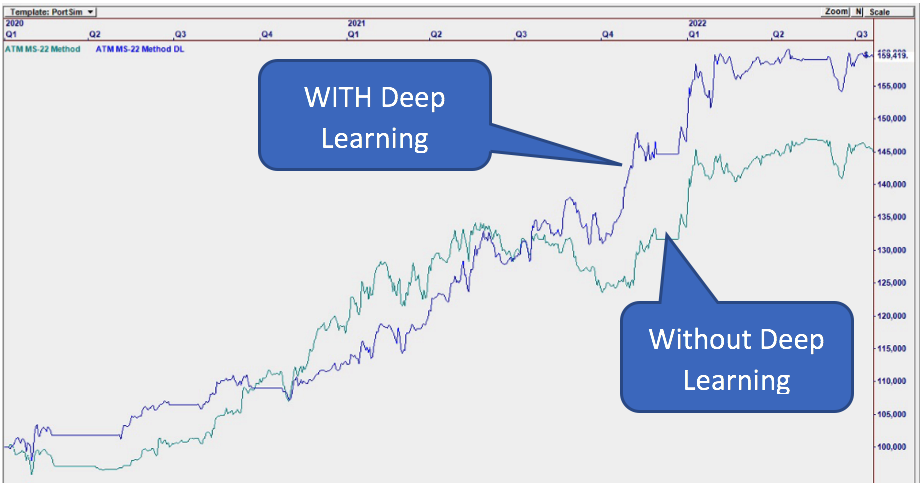
Improves Existing Strategies
Going from 68% Accurate to 83%
And QUADRUPLING Profit per Trade!
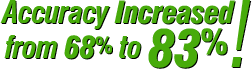
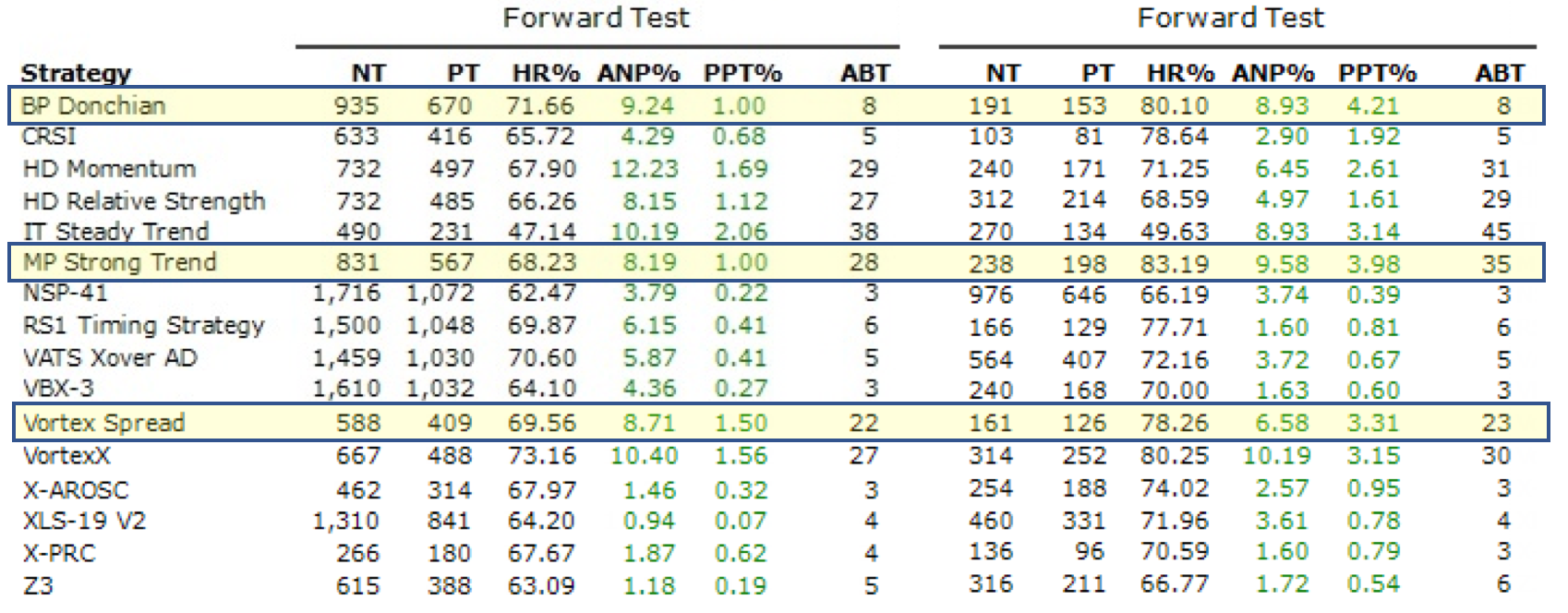
We applied Deep Learning to our most popular Plug-In Strategies.
While 98% of them were improved in the Forward Test, several were dramatically improved:
• BP Donchian Hit Rate went from 71% to 80% and PPT Quadrupled, going from 1% to 4.21%
• MP Strong Trend went from 68% to 83%, with PPT also Quadrupling (1% to 3.98%)
• Vortex Spread went from 69% to 78%. PPT more than Doubled from 1.5% to 3.31%
Mathematical Proof
…that Deep Learning Works.
The Deep Learning Module calculates a Score for every Signal. This plot shows the average
Hit Rate as Minimum Score is increased, demonstrating that higher Scores generate better Signals.
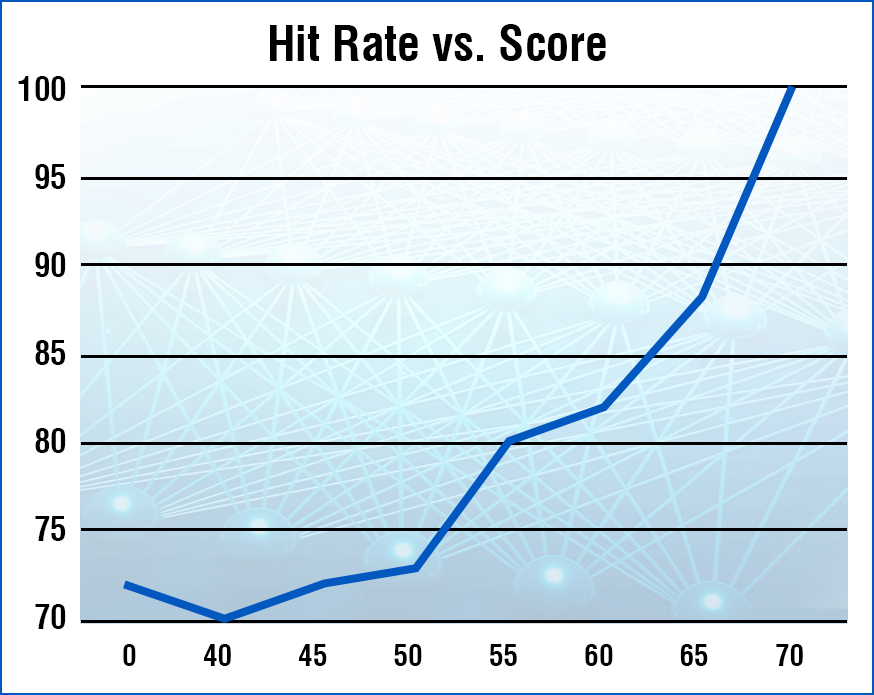
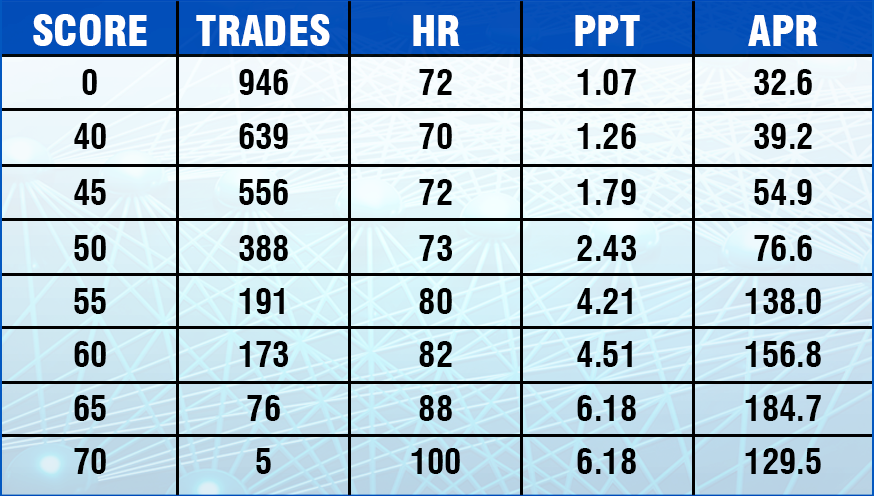
We see the same for Profit per Trade (PPT) and Annual Percentage Rate of Return (APR). CONCLUSION: The Deep Learning module knows which Signals are the most profitable.
A Simple RSI System Becomes a Winner
We added Deep Learning to a simple
RSI Reversal System.
Buy When:
Buy When:
• RSI is below the classic oversold level.
RSI(5) < 20
• RSI is reversing over 3 bars.
RSI(5)[2] > RSI(5)[1] and RSI(5) > RSI(5)[1]
A Default Trade Plan was used in the
Strategy. But for Training, the Target (Exit)
was set at 5 bars.
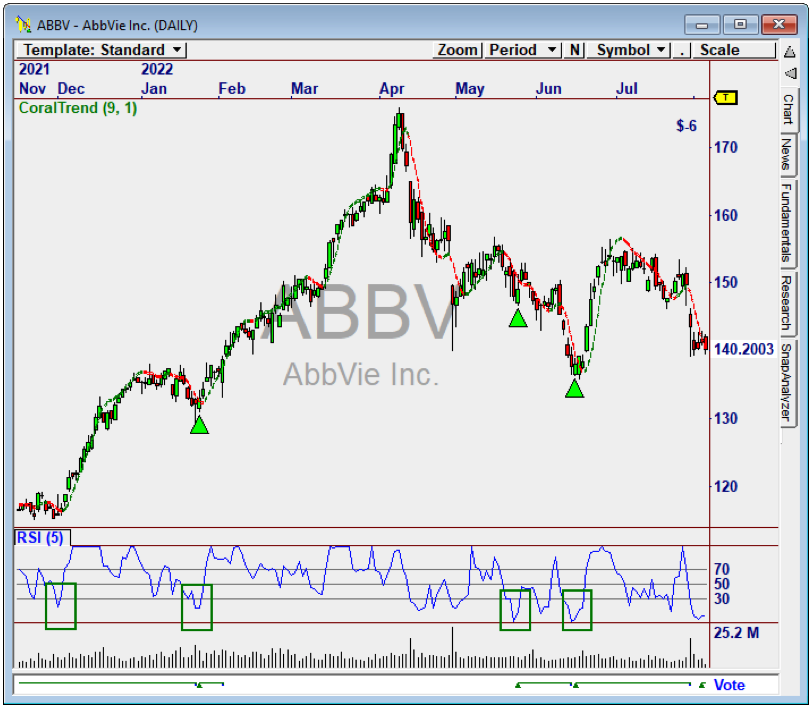
2 Steps
1. Drop the Block
into the Strategy.
2. And Re-Train.
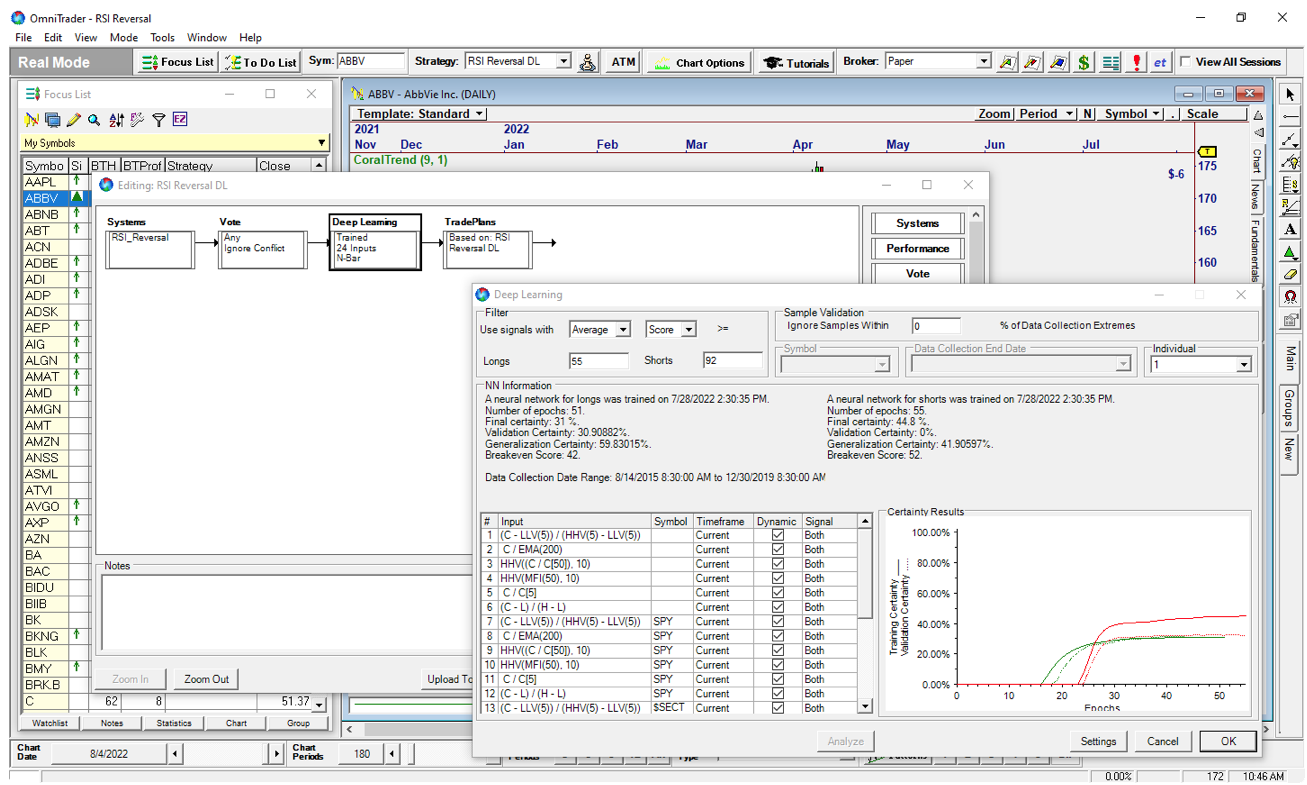
(Click Image Above for Higher Resolution)
The Deep Learning Block was able to improve this Simple Strategy.
The DL versions use a Deep Learning block. In the case of Longs and Shorts,
the difference in performance is fairly dramatic.
RSI Reversal With & Without DL:
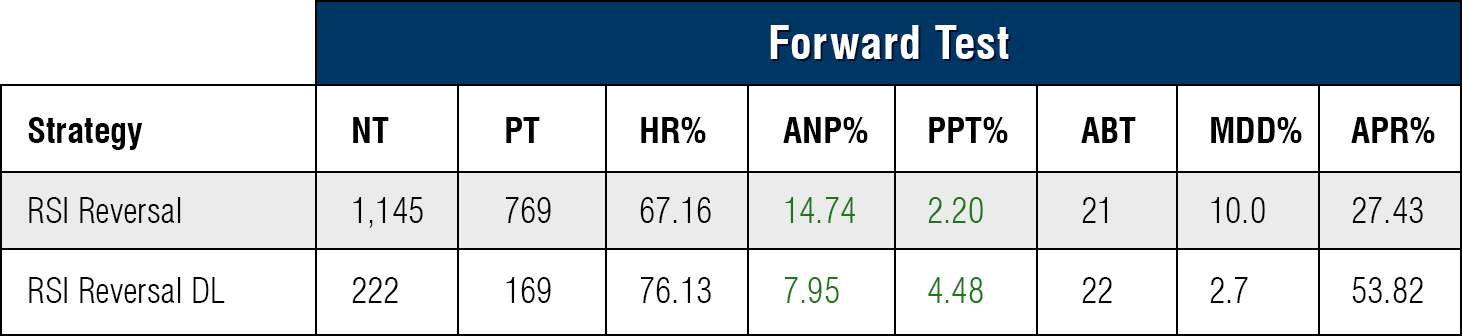
Note - For these statistics, RSI Reversal DL is set to 90 for Advisor Cutoff.
With Shorts Applied
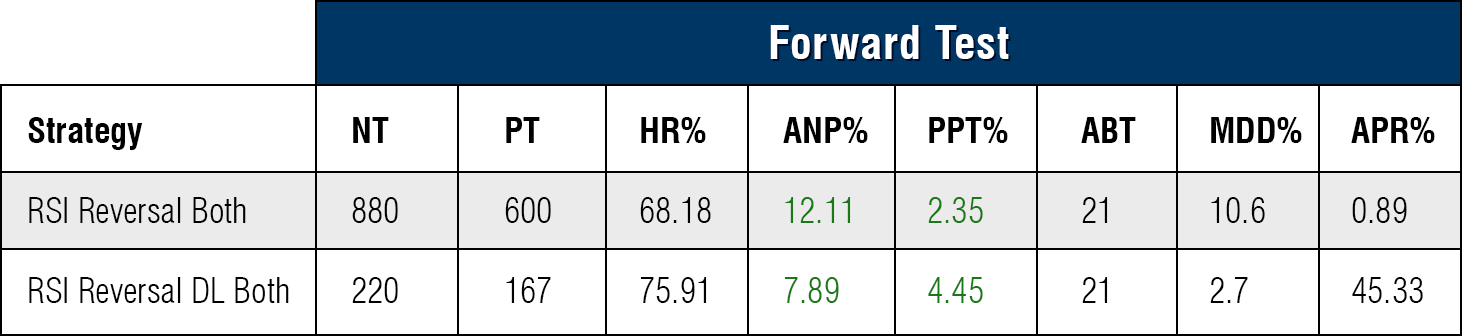
Note - For these statistics, RSI Reversal DL is set to 90 for Advisor Cutoff.
Think what Deep Learning could do with YOUR Strategies!
Recent Signals
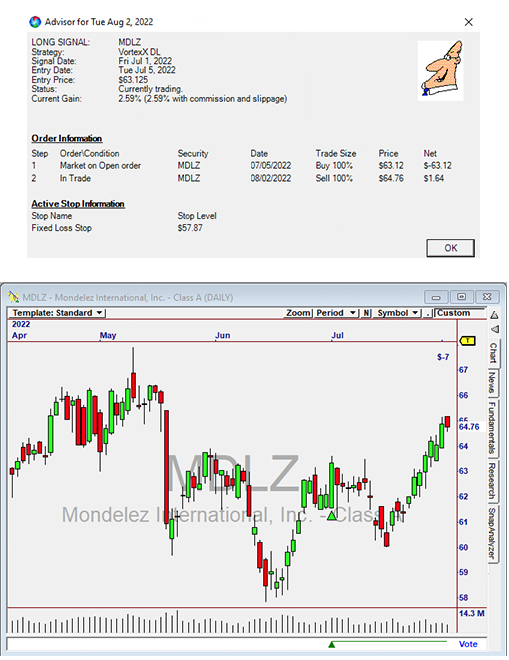
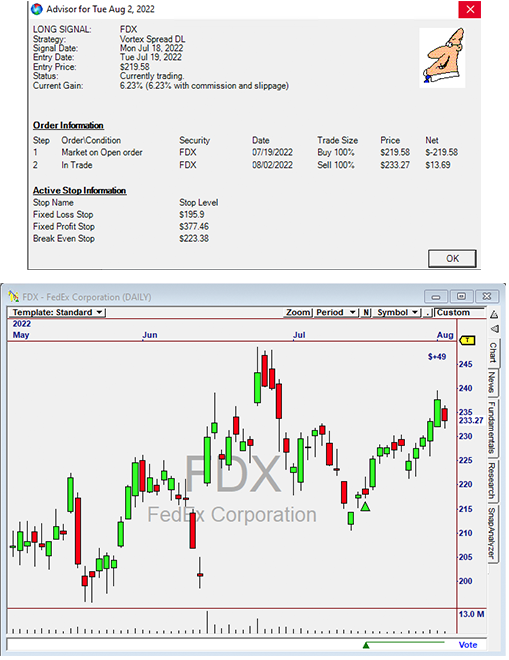
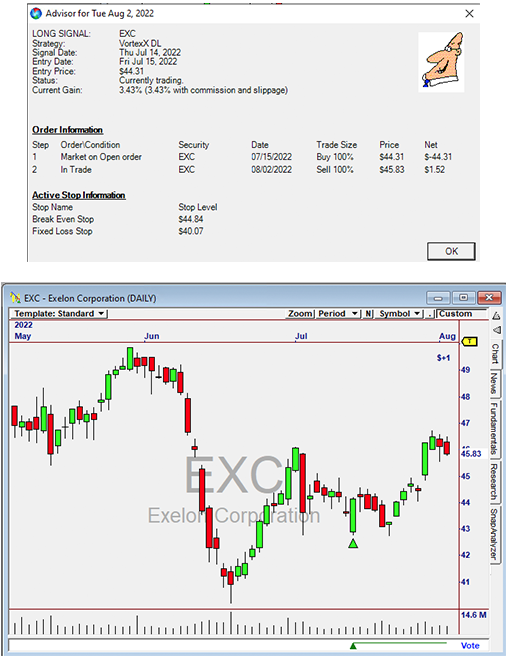
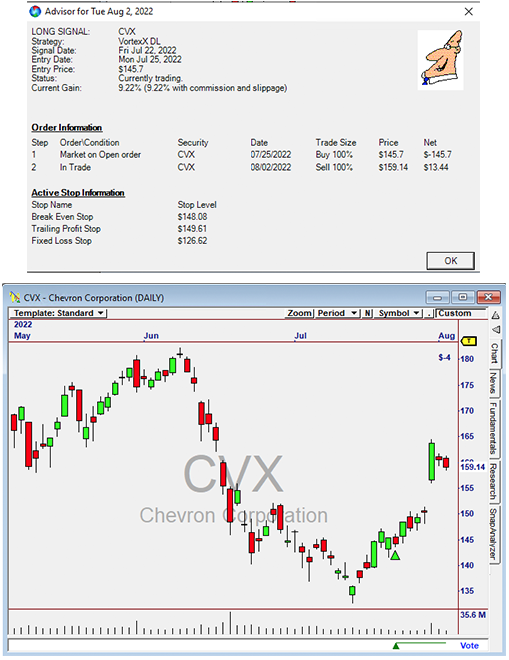
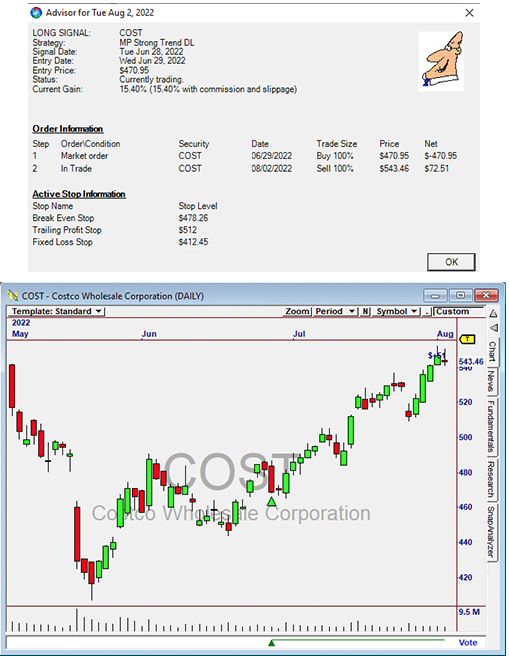
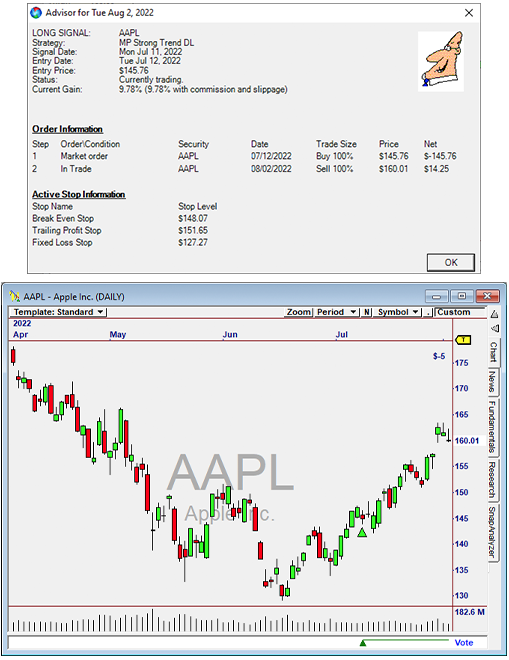
Get the Advantage of Deep Learning Now!
Here’s how you can get this amazing new A.I. Technology for Traders...
Nirvana Club Members:
Any Current Member can get
the latest version of OmniTrader.
Click Get My CD Keys in the Help-Registration
“A Nirvana Club Membership is my best trade
investment to date!” - Reece Beane
Join the Club!
The Nirvana Club was founded in 1996 for the purpose of developing advanced Strategies based on
Artificial Intelligence. Members have access to so many assets in The Club, including…
• The Genetic Algorithm Block – Finds Profitable Trading Rules in your Data.
• The Consensus Block – Finds relationships between Indicators.
• Many Great AI Strategies developed over 25 years, Ready to Download!
• Nirvana Club Forum – the Most Active Trading Community in the Industry.
• Great Product Discounts on Nirvana Software and Services.
• And More!
Click Here to get the complete Story including great videos on all the
Club has to offer, including a description of the many Club Benefits.
This comprehensive video explains the many benefits of a Nirvana Club membership, including:
* Exclusive A.I. Trading Technology
* Proprietary Products Created for Members
* The Amazing People in The Club
* Generous Member Benefits
* And more!
Nothing beats the incredible value of a Nirvana Club Membership!
Important Information:
Futures, options and securities trading has risk of loss and may not be suitable for all persons. No system can guarantee profits or freedom from loss. Past results are not necessarily indicative of future results. Hypothetical or simulated performance results have certain inherent limitations. Unlike an actual performance record, simulated results do not represent actual trading. Simulated trading programs in general are also subject to the fact that they are designed with the benefit of hindsight. No representation is being made that any account will achieve profits or losses similar to those shown.